Cloud – On-prem, Hosted/Managed, Public, Private, Edge
Databases - RDBMS and NoSQL
End User Computing (VDI and DaaS)
Expanding the Applications Running on HCI
Industry Solutions
Solutions Architecture
Dive in to the details for architecting solutions on Nutanix Cloud Platform. Nutanix Validated Designs, Reference Architectures, Best Practice Guides, and Tech Notes are available across a wide array of solutions to meet your specific needs.
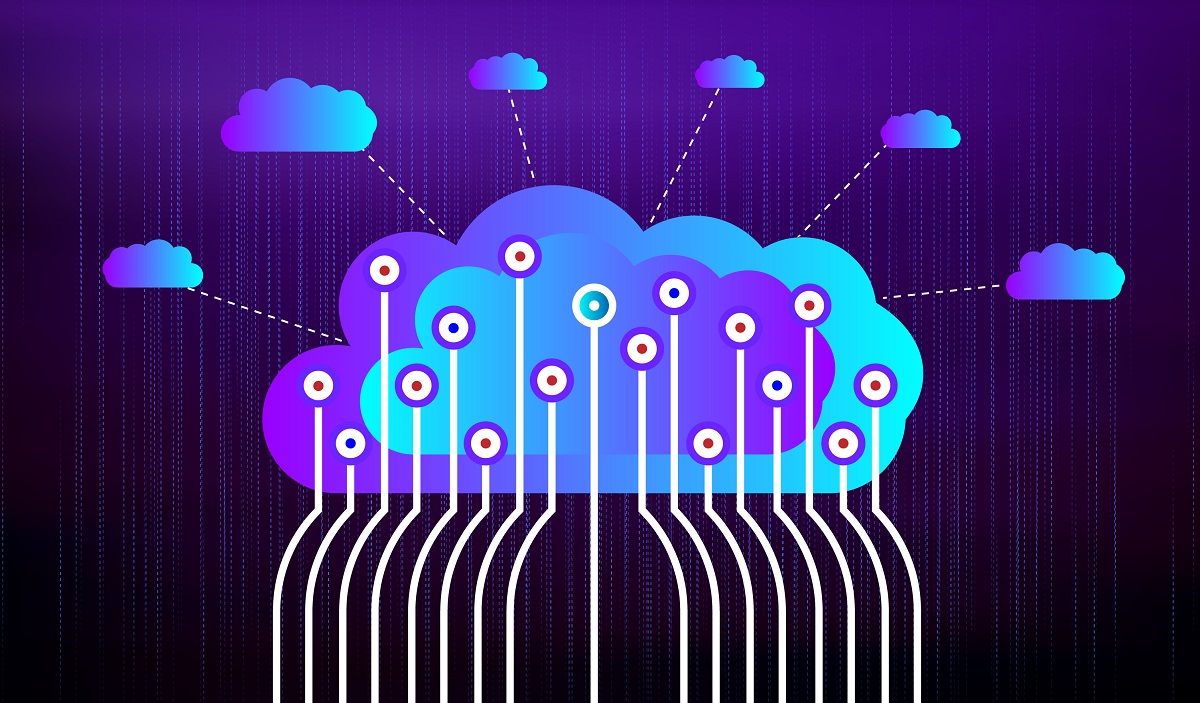