Many people start their days asking Alexa about the weather. They shoot out quick replies to emails with Gmail’s Smart Reply function. They drive to work using map apps that reroute each journey to best avoid traffic (and traffic cops). Once back home, they vegetate in front of the television as Netflix takes over control of entertainment needs.
Without even realizing it, the world is increasingly powered by AI. When asked if the world is better or worse since the widespread adoption of AI, 61% of respondents agreed in the affirmative, according to ARM’s Global Artificial Intelligence Survey.

While the previous version of this article cited a Gartner finding that businesses were slower to embrace AI than consumers, the tide has changed now. Well over 50% of companies have accelerated their AI adoption plans as a direct result of the COVID-19 crisis. And a reassuring 86% of companies said AI was a mainstream technology in business, the Harvard Business Review reported.
One of the most significant areas in which companies and organizations can start leveraging AI is decision-making, because it can process a lot more data and information than humans and identify patterns and trends in consumer behavior.
Enter predictive algorithmic forecasting.
What is Predictive Algorithmic Forecasting?
Any form of “forecasting” essentially paints a scenario about the future based on current and historical data.
Predictive algorithmic forecasting refers to a method of AI-based estimation, where statistical algorithms fed with historical data make predictions on what is likely to happen in the future. As more data flows into the algorithmic model, the model automatically “learns” more about the scenario and its predictions get increasingly more accurate with time.
Essentially, the algorithms take the output of predictive analytics run on existing data and use it as an input in their forecasting models. It is the 5th most common application of AI in business, a McKinsey study found.
Unlike traditional statistical forecasting, which gives one set of results and rests on them, predictive algorithmic forecasting is not static. It becomes more precise and course corrects automatically based on the data flowing into its model.
However, AI isn’t the only aspect that matters to a good predictive algorithmic model. The most successful predictive algorithms are those that have a strong human intelligence component to them. It takes an astute data scientist to translate the findings from a predictive algorithmic model, turn them into recommendations, and apply them to a business scenario.
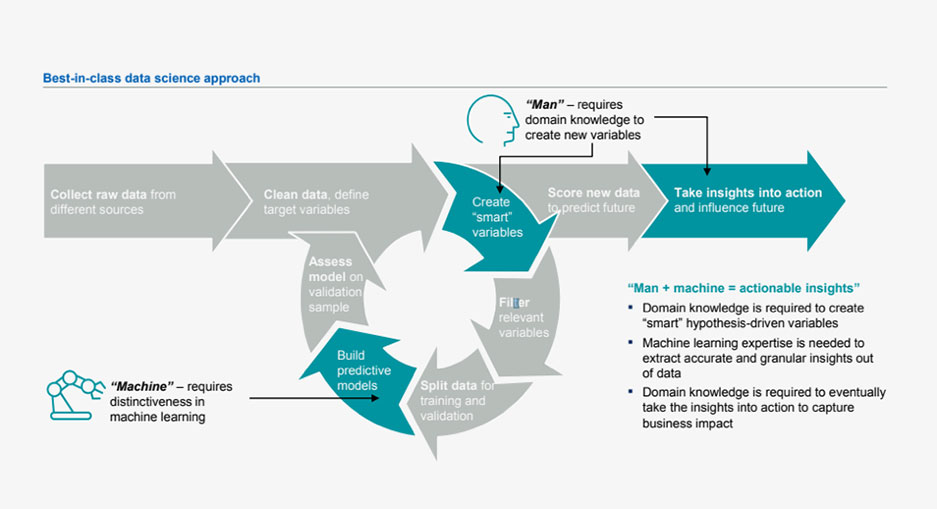
This combination of human and artificial intelligence is what delivers the 1-2 punch setting predictive algorithmic forecasting head and shoulders above its traditional counterparts.
The Importance of Predictive Analytics in Forecasting
So what’s driving businesses’ new-found love for predictive analytics and models as HBR, PwC, and others have found? In a nutshell, companies have recognized how predictive algorithms can give them a real competitive advantage, help differentiate their products and services, and boost their bottom lines.
Not that all businesses consciously realize this. “AI and predictive analytics is permeating your organization and you're not even necessarily aware of it,” said David Kuder, Principal at Deloitte and head of their Cognitive Insights & Engagement offering.
With rapid advances in consumer tech, increase in data collection and analysis, and low-code software, predictive analytics is no longer the domain of scientists and mathematicians. It is a function whose time has come.
The predictive analytics industry was worth $8 billion in 2020 and is projected to garner a total revenue of $39 billion by 2028, growing to nearly five times its current size. Increasingly, organizations are using predictive analytics and algorithms in more and more business functions, uncovering new opportunities and solving persistent problems in the process.
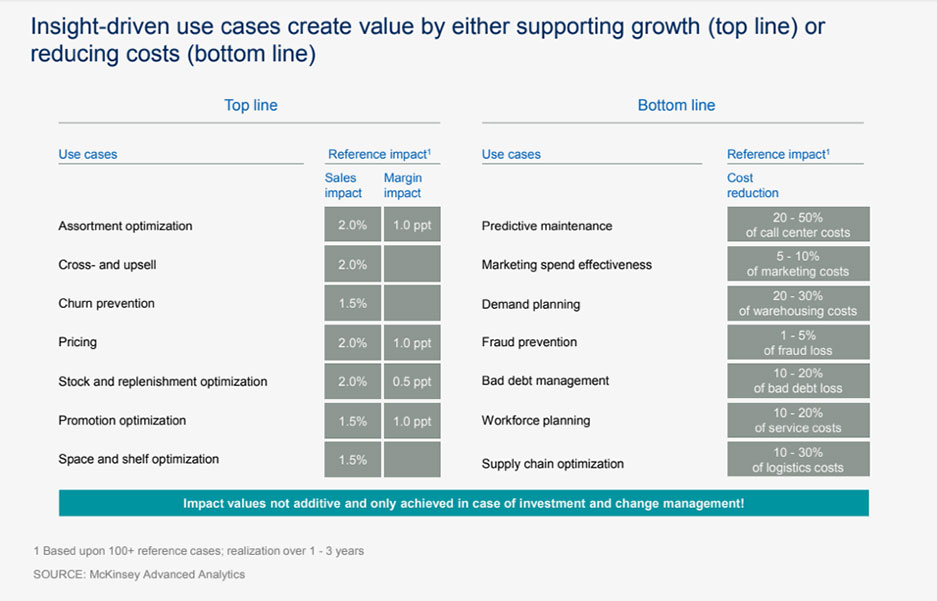
Businesses can use AI-based technology to create value in two ways: scaling up or saving costs. Nutanix Cost Governance is a case in point. AI-driven algorithms track customers’ cloud usage patterns, identify under-utilized resources, and proactively offer recommendations for cost savings.
Here are some common ways predictive analytics is helping organization meet industry-specific challenges.
Marketing
Easily the industry where predictive analytics – or any sort of analytics and algorithms – has made the greatest difference, marketing relies on (and makes use of) consumer data today much more than ever before. Everything from ad impressions to clicks to brand interactions to purchases are tracked by various “martech” tools.
Predictive modeling helps assimilate and integrate data stored in various datasets, make sense of the customer’s journey by forming a cohesive intent out of engagement on various channels, and finally score each prospect on the basis of how likely they are to purchase the product. The converse is true as well – algorithms can predict customer churn rates by analyzing signals that indicate individual customers are losing interest in a brand’s products.
Assisted by predictive algorithms that accurately gauge the customer’s propensity to buy, sales and marketing teams can prioritize leads to target and tailor their outreach to various audiences with content that’s relevant to them at that point of time.
All in all, an effective marketing and advertising strategy is no longer dependent on gut feeling or experience. If John Wanamaker was alive today, he wouldn’t have said, “Half the money I spend on advertising is wasted. The trouble is, I don't know which half.”
Higher Education
Predictive analytics have proved a blessing in disguise for school and university administrators, enabling them to accurately forecast students’ ability and inclination to complete a course of study.
Predictive algorithms can process students’ high school scores and predict their scores in university with a high degree of accuracy. This helps teachers and administrators support students in the best way possible with appropriate facilities. Just like marketers can minimize product churn, administrators can persuade students contemplating dropping out of their course or switching colleges to stay back at the school and finish their studies.
Retail
Analyzing customer behavior has a lot of implications in merchandising and planning for retailers. This is where predictive algorithms that process huge amounts of data accurately excel. Retailers can predict customer demand by channel, stock shelves at the appropriate times, cluster products together, and also plan replenishment strategies.
Taken online, the advantages to businesses are magnified many times, while the benefits to consumers are distributed on an individual level. Amazon’s personalized product recommendations laid the foundations for its massive, loyal user base, thanks to its collaborative and content-based filtering features.
Insights from predictive analytics can also help retailers tweak pricing and promotions according to forecasted demand, inventory lifecycle, and competitors’ tactics. Analysis of buying patterns allows retailers to estimate Customer Lifetime Value (CLV) of a given segment or cohort fairly accurately.
Logistics and Supply Chain Marketing
SCM is a business area which is perhaps the most susceptible to uncertainties and external factors (including economic, technical, and regulatory). A poorly optimized supply chain can spell doom for businesses – the negative impacts spill over into purchase, manufacturing, delivery, customer service, and just about everything else.
Predictive analytics makes sure every bit of real-time data is taken into account and every stakeholder throughout the chain has up-to-date information to “predict” what they will receive and when. This takes decision-making and process efficiencies to a whole new level. Management has the opportunity to evaluate more risk factors and variables and model every scenario to see how things can play out.
Banking and Finance
When huge amounts of money are at stake, it takes predictive algorithms to crunch an equal amount of numbers to control its flow. They don’t say “Data is the new oil” for nothing.
Large banks and financial institutions – and now, present-day “fintech” and “finserv” startups – routinely deal with massive amounts of personal information while processing loan, credit card, insurance, and other asset applications. Humans are ill-suited to assess and process this information at a large scale for extended periods of time. Predictive algorithms on the other hand have an error-free and empirical evidence-based way of judging whether to grant the request or go ahead with the transaction or not.
The finance industry was one of the first to adopt predictive analytics and machine learning (ML) to measure credit risk and predict and reduce loan defaults and frauds. Simultaneously, they identified, acquired, and retained more high-value, low-risk customers.
Examples from the Real World
Applications of predictive algorithms at work exist in almost every industry, including finance, healthcare, transportation and consumer goods.
These kinds of real-time analyses impact businesses’ bottom lines just as they touch lives and make the world a safer, better, and more convenient place.
Healthcare and Suicide Prevention
Researchers at the Vanderbilt University Medical Center used algorithmic forecasting powered by ML to create a suicide prediction model that could be applied to incoming patients at all hospitals.
They used hospital admissions data for over 5000 patients, including age, sex, zip code and diagnostic history to build a model that could predict the likelihood of a patient being suicidal. The model predicted the possibility of a suicide over the next week, in the case of new incoming patients with an 84% accuracy.
What’s more, it continued to be able to predict the probability of a patient’s likelihood to commit suicide up to two years down the line to 80% accuracy, thus helping hospital staff and doctors from reaching out and offering timely support to prevent such a drastic step.
Social Services and Improving the Foster Care System
ML algorithms were harnessed by the social entrepreneurship company DataKind in central Florida, to help foster care workers in the county improve their productivity and reduce caseworker turnover.
They built a tool that uses historical data to predict how many hours a new case will take. This helps in assigning the right number of cases to each case worker to optimize case load and prevent worker burnout.
It also helps case workers plan their activities for each week using past historical case data, maps and routing data from Bing Maps. Case workers can enter their case details into the tool and get an algorithmically-determined plan for their week, including meeting times, routes and visits without having to waste time planning, rerouting or canceled visits.
“I have seen route optimization make a 30-mile difference for a day’s worth of activities,” said Matt Baker, Director of Business Analytics and Automation at Community Based Care of Central Florida (CBCCF). “That can save hours a day and $2,025 a day in travel costs alone for 150 case managers.”
Insurance and Claims Processing Efficiency
Zurich Insurance uses predictive modeling and AI to automate a large part of its cumbersome injury claims filing and assessment process. The medical report evaluation process is fully automated; routine tasks like re-entering data into the system and updating databases are done by software, freeing up time for employees to focus on more value-added activities like negotiating settlement amounts with the claimants.
This combination of automation and AI tools has helped Zurich Insurance improve productivity without any impact on the quality of decision-making. Time taken to assess a medical report went from one hour to just a few seconds, saving nearly 40,000 hours of manual work and about $5 million in overall cost savings.
What’s Next?
Though we see the significant ways in which AI and algorithmic forecasting have changed the way we live and do business, somewhere there still exists a reluctance to consider AI as nothing more than a shiny new toy, whose real revenue impact is still nebulous.
However, a Deloitte study has proved this mindset to be unfounded. A staggering 83% of early adopters of AI have already achieved moderate (53%) or substantial (30%) economic benefits from their AI investments.
These returns are only set to leapfrog with time. Data from Accenture shows that AI and algorithmic forecasting will boost profitability by 38% and generate additional revenues to the tune of $14 trillion by 2035.
The real question now is, how many SMBs and enterprises will grab sizable chunks of that additional revenue by building predictive models and applying them in their everyday operations?
This is an updated version of an article originally published on October 17, 2019.
Dipti Parmar is a marketing consultant and contributing writer to Nutanix. She’s a columnist for major tech and business publications such as IDG’s CIO.com, Adobe’s CMO.com, Entrepreneur Mag, and Inc. Follow Dipti on Twitter @dipTparmar or connect with her on LinkedIn for little specks of gold-dust-insights.
© 2022 Nutanix, Inc. All rights reserved. For additional legal information, please go here.
Related Articles
Content is loading...