THOUGHT LEADERSHIP
Machine learning and artificial intelligence aren’t new concepts—they’ve been around for decades. But Hillery Hunter, Director of Accelerated Cognitive Infrastructure at the IBM Research Division, says that today we’re witnessing an incredible convergence of technologies that has created the perfect environment for ML and AI to take the stage.“It’s a confluence of the data and the compute abilities at the same time that has created this skyrocketing interest in machine learning.”
In the beginning, there was Big Data. And people were excited about the possibilities of what they could do and learn with all that information. We gained some insights and began to apply it to business processes—but quickly saw that the never-ending deluge of data was perhaps too big for enterprise computing technology at the time.
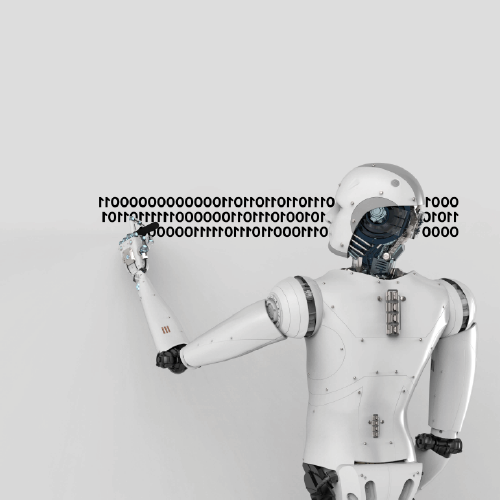
Meanwhile, researchers were developing techniques for massively parallel computing, using graphics processing units or GPUs. These GPUs empowered data scientists to crunch much larger amounts of data, much quicker, using fewer IT resources. Now it was possible for enterprises to get close to the same high-performance computing capabilities that were traditionally the sole domain of academic research institutions and government labs.
The explosion of information and the maturing of parallel computing technologies have enabled a whole new level of analysis and insights, which is still, at its heart, about the data. “That’s the bottom line,” says Hunter. “The data is the resource that everyone has and it’s where people want to try and make a difference for their business, be that improving consumer experiences or net profitability of the business or outcomes.”
In fact, Hunter says, ML is the technology that is finally fulfilling the promises made by Big Data more than a decade ago. It’s the key that is opening the door to new, exciting insights and possibilities for enterprises today.
Getting Down To Specifics: How ML Can Transform Your Business
As enterprises move more of their workloads to the cloud, it’s becoming critical to incorporate ML and AI technologies to deliver the immediate, personalized experiences customers want and provide employees with quick, easy access to data and applications around the clock.
“Machine learning capabilities should be natively integrated into the cloud stack—it’s the only way to deliver the same services without the need for an army of specialized IT resources,” says Maryam Sanglaji, Principal Product Marketing Manager at Nutanix.
But there are sweet, sweet rewards in store for those enterprises that integrate ML and AI technologies into their operations. Sanglaji says the three biggest enterprise benefits of machine learning are improving risk management; optimizing performance and spending; and simplifying operations.
Risk Management, Revitalized
Sniff out suspicious activity and take it down. ML is a master at detecting anomalies, which makes ita powerhouse for improving risk management. A ML algorithm in your system can monitor operations and come to recognize what’s normal and what’s not. When it sees an anomaly, such as a link failure due to network congestion, it can alert you immediately or, in many cases, take action to resolve the issue itself (that’s self healing). As the algorithm detects disruptions and automatically repairs them on the spot, your IT team has more time to focus on more critical business.
Become clairvoyant about security risks. A recent study showed that it takes businesses an average of 191 days to detect a data breach and another 66 days to contain it.* But with ML that knows your system’s baseline normal, it might be able to detect and contain that breach much sooner—costing you much less money in the end. A ML algorithm is able to aggregate all past and current data to see how and when attacks happen. With that knowledge, it can detect some illicit activities right as they begin and take steps to secure the system before the threat has time to fully develop. By recognizing attack patterns, the system can learn to predict oncoming threats by knowing the early steps it took last time.
Run the whole kit and caboodle. Almost a quarter (22%) of data center outages are due to plain old human error.† We’ve developed technology to automate many tasks to help reduce that number, but we can go much further with ML. With such a smart algorithmin your system already detecting anomalies, preventing security breaches, and proactively resolving issues before you even know about them, why not go all the way and have the ML program become the admin? A ML program could certainly learn to deploy environments and manage them, taking the human and the error out of the equation.
Optimization-Business Jargon For Being At Your Best
Put data in its place and keep it safe. Where you store data in the data center matters. ML can learn how, where, and when the data is used and accessed and then place that data in the best place possible. It also has deep insight into the hardware and software your data runs on, so it can move data around if it detects potential failure of a hardware component, for instance. ML is great at monitoring who accesses data and when, and creating a baseline of normal so it knows when someone is attempting to access data outside of the norm. ML can also create security policies on the fly as it detects unauthorized activity outside of the pattern. In that senseit’s extremely proactive.
Make peak performance the new normal. Things have to work when customers use them. No one likes calling customer service and being put on hold because the system is slow. When a customer signs into your service, it better be working at its peak or you could lose business. All of the ML abilities mentioned above can help you in this area. Putting data in the right place, making access easy, detecting anomalies and resolving them before they escalate, protecting the system from security breaches—they all add up to the consistent, reliable user experience that keeps customers coming back.
Go green to save green. Not only can ML keep your data and systems running at their peak, it can also ensure that the data center environment itself is optimal. Thanks to sensor technology, ML can monitor every device in the data center and save energy by powering down unused devices. This can save you a lot of money and make your data center more energy-efficient as well. In fact, ML can become the brain of a smart building for even greater energy efficiency, managing heating, cooling, lighting, and more.
"In a big way, ML is the technology that is finally fulfilling the promise made by big data more than a decade ago."
Operation Made E-Z
Create APIs that can strategize. A step beyond traditional APIs (which allow various software components to work together), ML-driven intelligent APIs give you more information about the environment the software is in and the application users. So not only do they serve their core purpose, they can also alert you to relevant situations, like running out of capacity for that application, for instance. Or consider an API plugin that can also give you information about specific customers or what forms of communication they prefer—or whether they’ve recently looked for a competing product. Intelligent APIs can enable machines to make strategic recommendations. Machines can tell another machine how to change and improve operations in very specific ways.
Run it all with just a few clicks. At the heart of it, this is about delivering simplicity. Streamlining processes and creating one-click type of operations. ML can eliminate the need to configure apps and devices separately and have specialized IT resources doing disparate tasks. It can remove complexity and manage that configuration and deployment, automated tasks that used to take a team of humans.
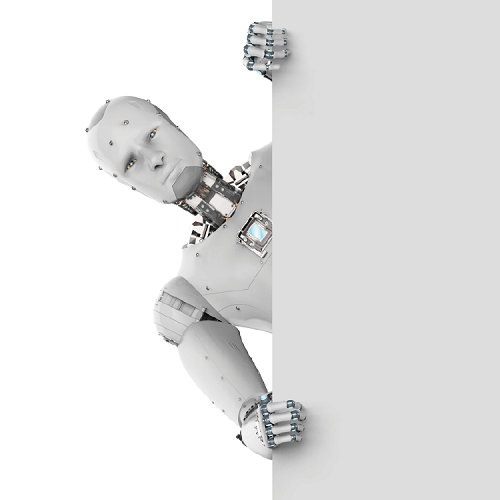
"Organizations that don't think ML applies to them can rest assured that their competitors are finding ways to use ML to improve their products, create better customer experiences, and increase their revenue."
GPUs Make Quick Work Of ML
Data scientists are using GPUs to accelerate compute times for ML. GPUs can drastically shorten experiment times—todays instead of months, hours instead of days, and minutes instead of hours.
An easy way to understand the difference between a GPU and a CPU (traditional computer processor) is to look at how they process tasks. A CPU has several cores that process tasks serially, one after the other—while a GPU has a massively parallel architecture made up of thousands of tiny, efficient cores that all handle their multiple tasks simultaneously. The end result is superfast operations.
Source: NVIDIA
Additional Content
Article
AI Will Improve Your Business and the Lives of Your Team Members
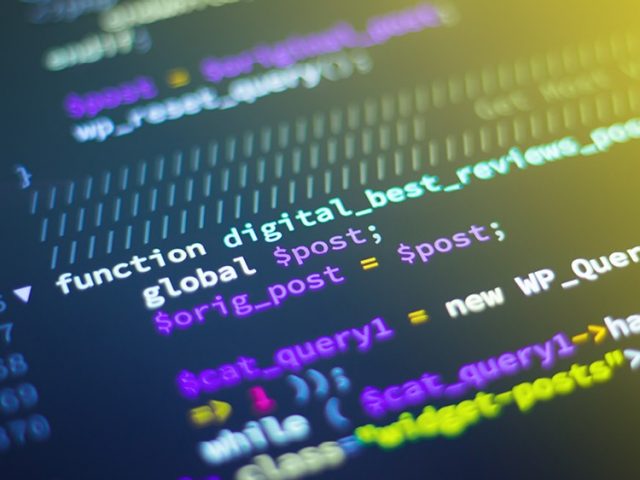